
The UK has been at the forefront of several strands of work that have made KGs as successful as they are today, in areas like: knowledge representation formalisms natural language processing machine learning methodologies to construct, learn and manage ontologies and knowledge bases de facto standard ontologies and vocabularies scalable reasoning, linked data etc. These include DBpedia, Wikidata, WordNet, Geonames, etc. Other knowledge graphs are openly available. The most common example is the Google knowledge graph, which is used in web search, or Amazon’s product graph. Some knowledge graphs are used primarily within the organisation that created them. They, including their schemas, evolve to reflect changes in the domain and new data is added to the graph as it becomes available. Alive: knowledge graphs are flexible in terms of the types of data and schemas they can support.This means that the graph is both a place to organise and store data, and to reason what it is about and derive new information. Semantic: the meaning of the data is encoded for programmatic use in an ontology, which describes the types of entities in the graph and their characteristics and can be represented as a schema sub-graph.

This makes it easy to integrate new datasets and formats and supports exploration by navigating from one part of the graph to the other through links. Graphs: unlike knowledge bases, the content of KGs is organised as a graph, where nodes (entities of interest and their types), relationships between and attributes of the nodes are equally important.While there are many definitions of knowledge graphs around, most of them agree that knowledge graphs are:

Knowledge graphs can be created from scratch, e.g., by domain experts, learned from unstructured or semi-structured data sources, or assembled from existing knowledge graphs, typically aided by various semi-automatic or automated data validation and integration mechanisms.
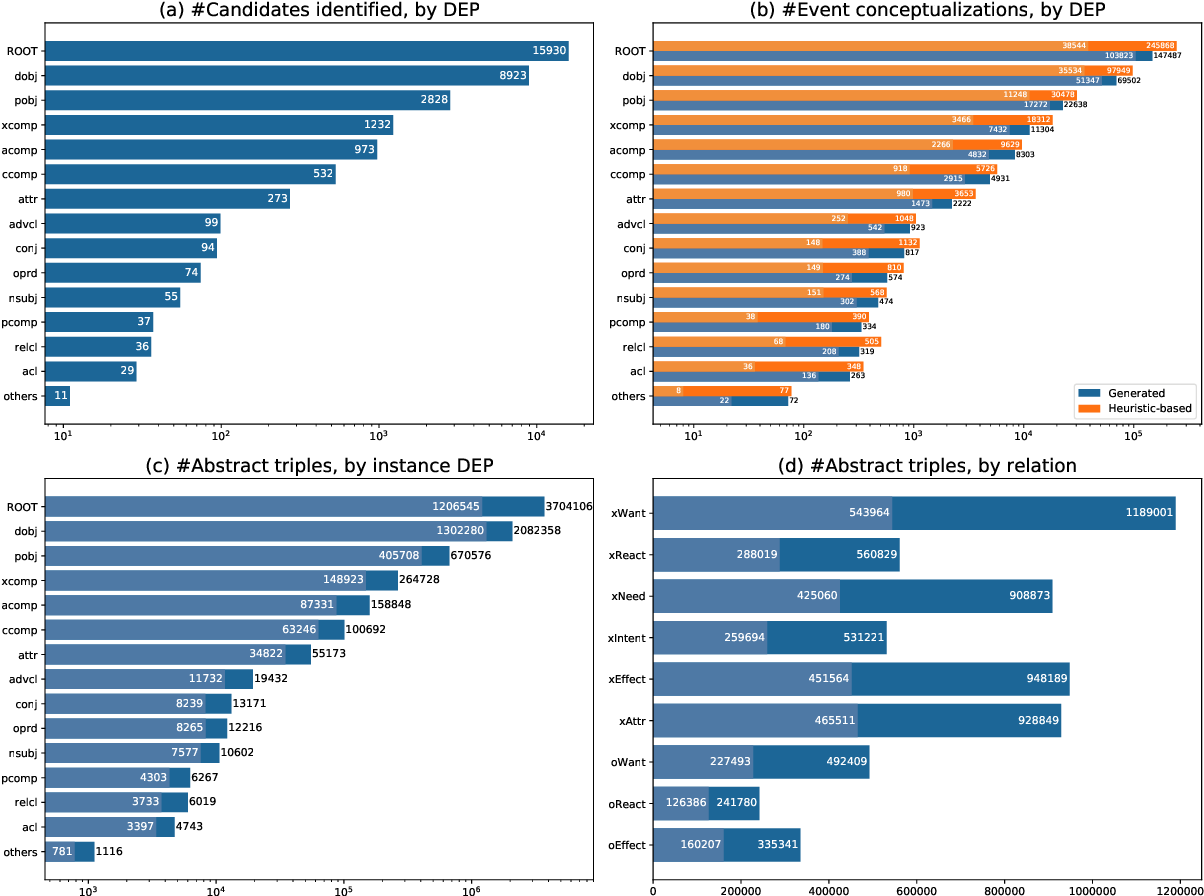
The term ‘knowledge graph’ has been introduced by Google in 2012 to refer to its general-purpose knowledge base, though similar approaches have been around since the beginning of modern AI in areas such as knowledge representation, knowledge acquisition, natural language processing, ontology engineering and the semantic web. Current partnerships and collaborations.
